Transform Your Data Recognizing With Second Dimensions
Exploring information via the lens of additional dimensions opens a world of untapped understandings, using a much more nuanced perspective on the complexities of your dataset. By deciphering the layers beneath the surface area metrics, you can discover patterns and connections that may have or else gone undetected, paving the way for educated decision-making and critical optimizations. This calculated usage of secondary measurements not just enhances your data understanding yet likewise acts as a driver for unlocking the full possibility of your analytics ventures.
Benefits of Secondary Dimensions
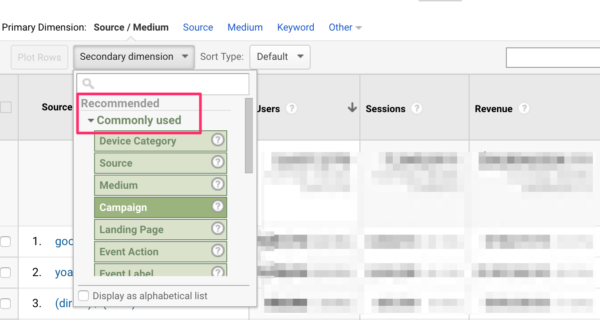
Secondary dimensions additionally allow organizations to conduct more detailed performance examinations. By layering additional measurements onto existing information, organizations can evaluate the influence of various variables on essential metrics, aiding them make more informed choices. Moreover, secondary dimensions help with the identification of brand-new chances for optimization and development by discovering partnerships that might have otherwise gone unnoticed. Overall, the utilization of secondary dimensions leads to more robust and insightful data evaluation, encouraging businesses to drive strategic activities based upon a much deeper understanding of their information.

How to Implement Additional Dimensions
To effectively implement secondary measurements in data evaluation, organizations should first identify crucial variables that align with their logical objectives and purposes. It is essential to take into consideration exactly how these second dimensions will certainly give extra context and deepness to the main data being analyzed.
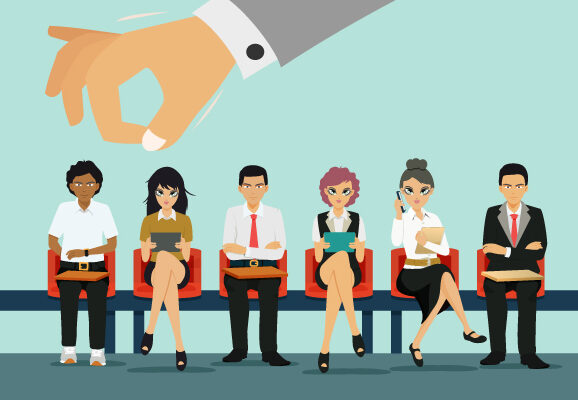
Studying Data With Secondary Dimensions
Making use of second measurements in information analysis enhances the deepness and context of understandings originated from key information. By including second measurements right into your analysis, you can gain an extra thorough understanding of the connections and patterns within your data. This procedure includes taking a look at the primary information with different lenses or point of views, which pop over to these guys can reveal hidden relationships or patterns that might not be instantly apparent when examining the data making use of only primary measurements.
Analyzing information with secondary dimensions permits you to segment and team your information in different means, supplying a more nuanced sight of your dataset. secondary dimensions. This segmentation visit this page can aid you determine specific aspects that may be influencing the end results you are examining. By drilling down right into the data making use of additional measurements, you can uncover beneficial insights that can guide decision-making and method advancement
Ideal Practices for Additional Dimensions
When including secondary dimensions into data evaluation, accuracy in specifying the measurements is crucial for extracting meaningful insights. It is vital to pick second measurements that enhance the key data effectively.
Another best practice is to stay clear of redundancy in dimensions. Make sure that the second measurements include brand-new point of views or information to the analysis, instead of duplicating information already present in the key measurements. This will certainly aid stop complication and enhance the interpretation of the data.
Additionally, it is essential to consider the scalability of the analysis when selecting second measurements. Pick measurements that can be quickly broadened or readjusted as required to fit future data demands or changes in analytical focus. By following these best techniques, experts can make best use of the worth of additional dimensions in information evaluation and gain deeper insights into their datasets.
Optimizing Insights Via Second Measurements
Incorporating secondary measurements purposefully improves data analysis by giving a much deeper understanding of the relationships within the dataset (secondary dimensions). By maximizing insights with secondary measurements, analysts can uncover valuable patterns, patterns, and dependencies that might not be quickly obvious when analyzing the information via primary measurements alone
One key benefit of utilizing second dimensions is the capability to sector and filter information more specifically. This segmentation permits a more granular analysis of details subsets within the dataset, making it possible for analysts to determine relationships and causations that may our website have or else been overlooked.
Additionally, second dimensions can aid in contextualizing primary data factors by adding layers of info that supply a more thorough view of the information. This contextualization is important for making educated decisions based on a holistic understanding of the dataset.
Verdict
In conclusion, including secondary measurements in information analysis processes uses a much more comprehensive and nuanced understanding of info, leading to enhanced insights and tactical decision-making. By incorporating extra variables that align with logical objectives, concealed correlations and trends can be exposed, supplying an extra thorough and contextualized sight of information. This strategy maximizes the potential for optimization and uncovers new opportunities within operations.
In general, the usage of secondary dimensions leads to more informative and durable information evaluation, equipping services to drive tactical actions based on a deeper understanding of their information.
Using additional dimensions in data evaluation boosts the deepness and context of insights derived from key information.Examining data with secondary dimensions permits you to sector and group your data in numerous methods, supplying a more nuanced sight of your dataset.When including additional dimensions right into information analysis, precision in specifying the dimensions is important for drawing out meaningful insights. Make certain that the second measurements add new point of views or information to the analysis, instead than replicating information currently present in the key measurements.